In a groundbreaking achievement, Google DeepMind, a subsidiary of Alphabet (GOOGL), has harnessed the power of artificial intelligence (AI) to predict the structures of over 2 million new materials. This milestone, published in the prestigious science journal Nature, holds the potential to reshape various industries by significantly improving the production of batteries, solar panels, and computer chips.
Traditionally, the discovery and synthesis of new materials have been both costly and time-consuming, often spanning a decade or more. However, DeepMind’s innovative AI, trained on data from the Materials Project, an international research group founded in 2011, has managed to predict the structure of nearly 400,000 hypothetical material designs. This breakthrough is expected to shorten the typically lengthy 10 to 20-year timeline for material development.
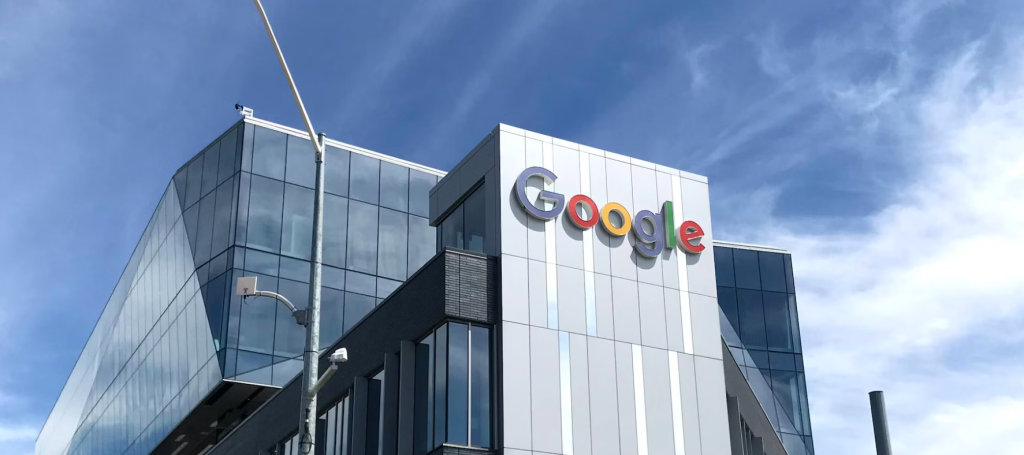
The GNoME Deep Learning Tool, a crucial component of DeepMind’s arsenal, identified an astounding 2.2 million new inorganic crystals, with 380,000 identified as the most stable for experimental research. This predictive accuracy extends to the stability of crystal structures, exemplified by the discovery of 52,000 new layered compounds similar to graphene and 528 potential lithium-ion conductors, a remarkable 25 times more than previous studies.
To facilitate further advancements, DeepMind is taking a collaborative approach by sharing its vast dataset with the research community through the Next Gen Materials Project. The data encompasses all of GNoME’s discoveries and predictions, providing researchers with free access to explore and experiment with the newfound treasure trove of material structures.
A significant leap towards efficient material synthesis has been made possible through DeepMind’s collaboration with the Berkeley Lab, resulting in the creation of a robotic laboratory. This autonomous lab successfully synthesized 41 of the newly discovered materials, demonstrating the transformative potential of AI in experimental synthesis.
Ekin Dogus Cubuk, a research scientist at DeepMind, expressed hope for substantial improvements in experimentation, autonomous synthesis, and machine learning models. The shared optimism extends to Kristin Persson, director of the Materials Project, who emphasized the need to shrink timelines for material development. Despite industry tendencies to be cautious about cost increases, the collaborative breakthroughs driven by AI may reshape dynamics, potentially reducing the time it takes for new materials to become cost-effective.
The convergence of AI and material science showcased by DeepMind’s achievement represents a radical acceleration in technological development. The impact on industries such as energy storage, electronics, and beyond is poised to unlock untold paths of innovation.
Related:
- Forecasting the Future: NASA and IBM Join Forces for AI in Weather
- China is Suffering from Lack of AI Experts Amidst Growing Artificial Intelligence Industries
- OpenAI Drama Unfolds Amidst AGI Progress and Safety Concerns
(via)