Apple‘s research team has taken a huge step forward with their new “MM1” multi-modal large language model. This exciting development was detailed in a recent paper titled “MM1: Methods, Analysis & Insights from Multimodal LLM Pre-training”, and it showcases a model with impressive capabilities in both image recognition and natural language reasoning.
The model is available in 3 billion, 7 billion and 30 billion parameter sizes
MM1 comes in three sizes: 3 billion, 7 billion, and 30 billion parameters. Researchers used these models to conduct experiments, pinpointing the key factors that influence performance. Interestingly, image resolution and the number of image tags have a greater impact than visual language connectors, and different pre-training data sets can significantly affect the model’s effectiveness.
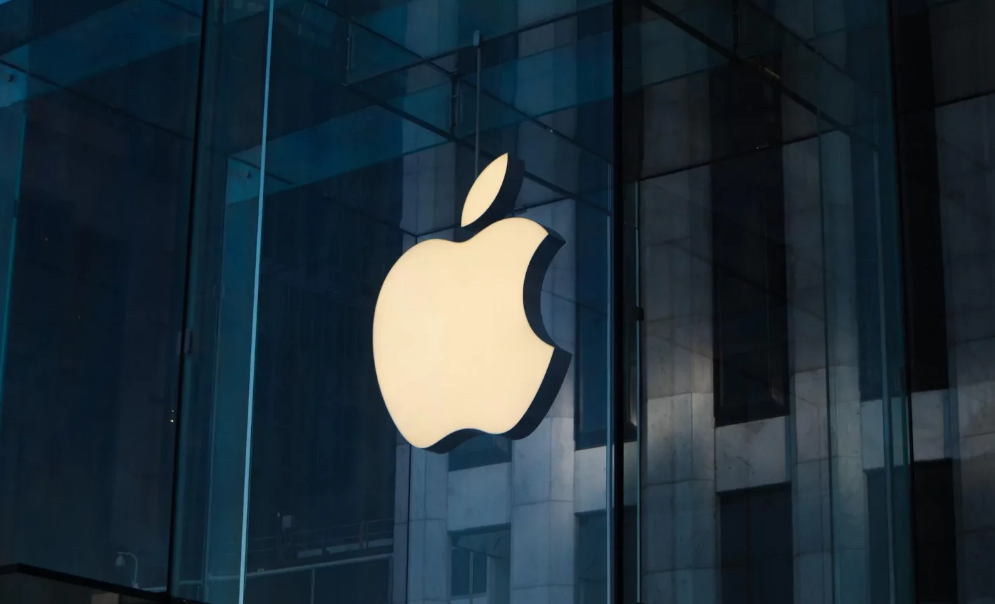
The research team meticulously built MM1 using a “Mixture of Experts” architecture and a “Top-2 Gating” method. This approach not only yielded excellent results in pre-training benchmarks, but also translated to strong performance on existing multi-modal benchmarks. Even after fine-tuning for specific tasks, MM1 models maintained competitive performance.
Testing revealed that the MM1-3B-Chat and MM1-7B-Chat models outperform most similarly sized competitors in the market. These models particularly shine in tasks like VQAv2 (question answering based on an image and text), TextVQA (text-based question answering about an image), and ScienceQA (scientific question answering). However, the overall performance of MM1 doesn’t quite surpass Google’s Gemini or OpenAI‘s GPT-4V models (yet). While MM1 may not be the absolute leader yet, it still is a significant leap forward for Apple in artificial intelligence. The company also recently acquired DarwinAI, read more about that here.
RELATED:
- Apple Acquires DarwinAI, Expect Lots of Artificial Intelligence-powered Features in the Future
- Apple may Launch an OLED iPad Air in 2028 and a Foldable iPhone in 2026 as Per Reports
- Get $100 OFF on Xiaomi 14 Pro at Giztop (1TB Variant)
- Lenovo Legion Y700 2023: Save $100 on this 8-inch gaming Android tablet
- How to turn off any Samsung phone without using screen (5 methods)
(VIA)